Conditionals
Contents
Conditionals#
from datascience import *
from cs104 import *
import numpy as np
%matplotlib inline
1. Booleans and Comparison Operators#
Let’s review a bunch of expressions that yield a True/False (or Boolean) result.
3 > 1
True
type(3 > 1)
bool
3 = 3
Cell In[5], line 1
3 = 3
^
SyntaxError: cannot assign to literal
3 == 3
True
x = 5
y = 12
x == 7
False
y - x
7
4 < y - x <= 6
False
4 < y - x
True
We can combine Booleans with and
, or
and not
to create longer logical expressions.
y - x <= 6
False
x == 5 and y == 12
True
x == 5 and y == 11
False
x == 5 or y == 11
True
x == 5 and (y == 11 or y == 12)
True
not True
False
not False
True
not not True
True
Broadcasting Comparisons#
Comparison operations can be used in array broadcasting too! We’ll use data about the game Monopoly to illustrate how.
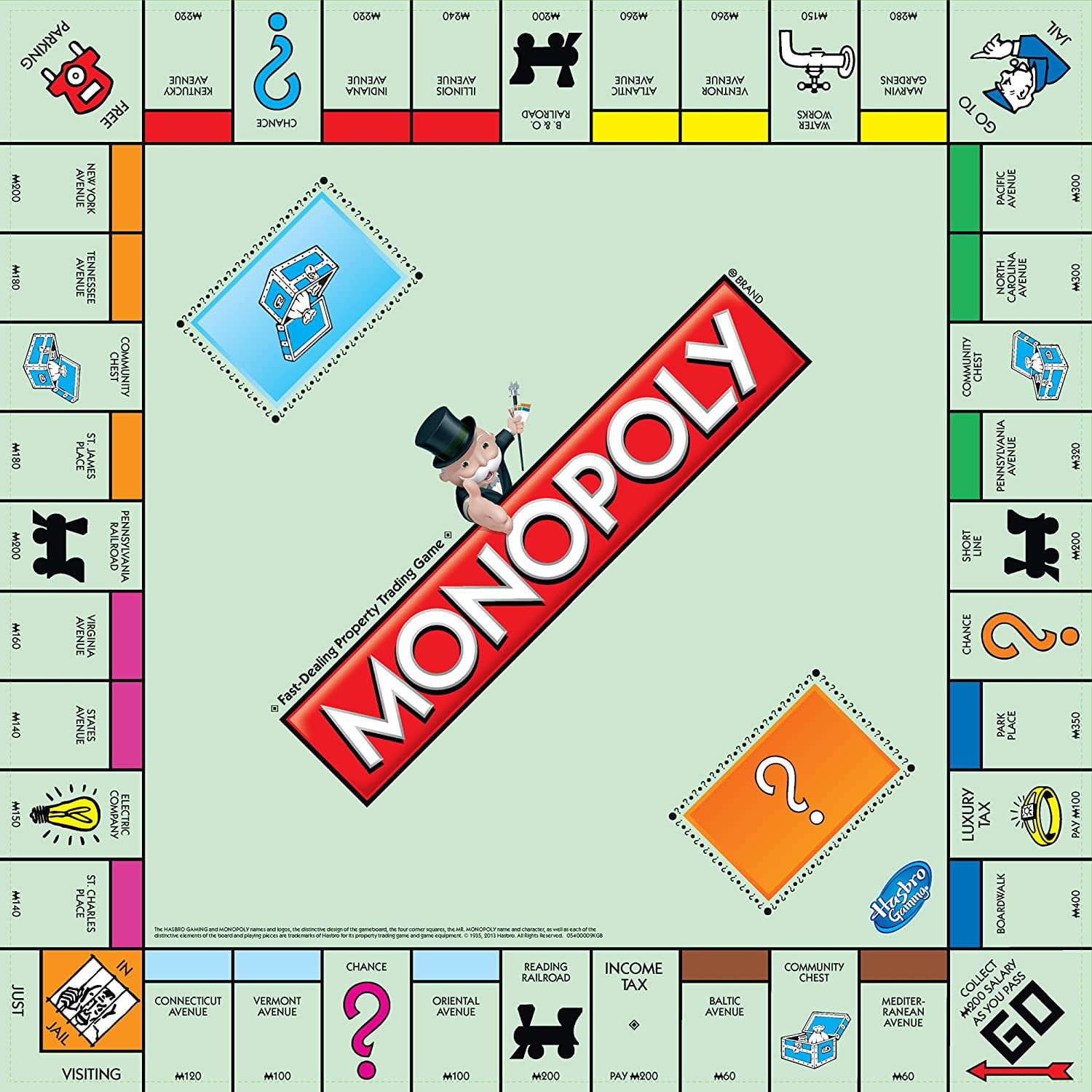
monopoly = Table().read_table("data/monopoly.csv")
monopoly
Name | Space | Color | Position | Price | PriceBuild | Rent | RentBuild1 | RentBuild2 | RentBuild3 | RentBuild4 | RentBuild5 | Number |
---|---|---|---|---|---|---|---|---|---|---|---|---|
Go | Go | nan | 0 | 0 | 0 | 0 | 0 | 0 | 0 | 0 | 0 | 0 |
Mediterranean Avenue | Street | Brown | 1 | 60 | 50 | 2 | 10 | 30 | 90 | 160 | 250 | 2 |
Community Chest | Chest | nan | 2 | 0 | 0 | 0 | 0 | 0 | 0 | 0 | 0 | 0 |
Baltic Avenue | Street | Brown | 3 | 60 | 50 | 4 | 20 | 60 | 180 | 320 | 450 | 2 |
Income Tax | Tax | nan | 4 | 200 | 0 | 200 | 0 | 0 | 0 | 0 | 0 | 0 |
Reading Railroad | Railroad | nan | 5 | 200 | 0 | 25 | 0 | 0 | 0 | 0 | 0 | 0 |
Oriental Avenue | Street | LightBlue | 6 | 100 | 50 | 6 | 30 | 90 | 270 | 400 | 550 | 3 |
Chance | Chance | nan | 7 | 0 | 0 | 0 | 0 | 0 | 0 | 0 | 0 | 0 |
Vermont Avenue | Street | LightBlue | 8 | 100 | 50 | 6 | 30 | 90 | 270 | 400 | 550 | 3 |
Connecticut Avenue | Street | LightBlue | 9 | 120 | 50 | 8 | 40 | 100 | 300 | 450 | 600 | 3 |
... (30 rows omitted)
Let’s focus on a subset of this data that just includes the name, color, and price of all the regular properties in the game.
tiny_monopoly = monopoly.where('Color', are.not_equal_to('None'))
tiny_monopoly = tiny_monopoly.where('Space', are.containing('Street'))
tiny_monopoly = tiny_monopoly.select('Name', 'Color', 'Price')
tiny_monopoly = tiny_monopoly.sort('Name')
tiny_monopoly.show(10)
Name | Color | Price |
---|---|---|
Atlantic Avenue | Yellow | 260 |
Baltic Avenue | Brown | 60 |
Boardwalk | Blue | 400 |
Connecticut Avenue | LightBlue | 120 |
Illinois Avenue | Red | 240 |
Indiana Avenue | Red | 220 |
Kentucky Avenue | Red | 220 |
Marvin Gardens | Yellow | 280 |
Mediterranean Avenue | Brown | 60 |
New York Avenue | Orange | 200 |
... (12 rows omitted)
Suppose we only have 220 dollars. How many properties could we buy?
price = tiny_monopoly.column("Price")
price
array([260, 60, 400, 120, 240, 220, 220, 280, 60, 200, 300, 100, 300,
350, 320, 140, 180, 140, 180, 260, 100, 160])
Let’s broadcast a comparison across this array. Does the type of the result make sense to you?
price <= 220
array([False, True, False, True, False, True, True, False, True,
True, False, True, False, False, False, True, True, True,
True, False, True, True])
np.count_nonzero(price <= 220)
13
How many properties could we buy for exactly 220 dollars?
price == 220
array([False, False, False, False, False, True, True, False, False,
False, False, False, False, False, False, False, False, False,
False, False, False, False])
np.count_nonzero(price == 220)
2
How many of the Monopoly spaces are light blue?
np.count_nonzero(monopoly.column("Color") == "LightBlue")
3
Small digression. There are limits to what we can do when using comparision operators and arrays… You may see errors like the following if you try to combine comparisons and broadcasting in seemingly-intuitive but unsupported ways:
10 <= price <= 400
---------------------------------------------------------------------------
ValueError Traceback (most recent call last)
Cell In[29], line 1
----> 1 10 <= price <= 400
ValueError: The truth value of an array with more than one element is ambiguous. Use a.any() or a.all()
We’ll try to steer you away from such cases, but if they occur, rephrasing as the following usually does the trick.
np.all(10 <= price) and np.all(price <= 400)
True
2. If Statements#
We can use if
statements to dictate which portion of our code should run based on Boolean expressions.
property_price = 50
if property_price < 100:
print("Inexpensive")
Inexpensive
property_price = 500
if property_price < 100:
print("Inexpensive")
property_price = 500
if property_price < 100:
print("Inexpensive")
else:
print("Expensive")
Expensive
Let’s use some best practices in computing and write a function for when we are re-writing the same chunks of code multiple times.
def price_rating(price):
"""Prints whether the price is
considered expensive or inexpensive"""
if price < 100:
print("Inexpensive")
else:
print("Expensive")
price_rating(500)
Expensive
price_rating(50)
Inexpensive
price_rating("blah")
---------------------------------------------------------------------------
TypeError Traceback (most recent call last)
Cell In[37], line 1
----> 1 price_rating("blah")
Cell In[34], line 4, in price_rating(price)
1 def price_rating(price):
2 """Prints whether the price is
3 considered expensive or inexpensive"""
----> 4 if price < 100:
5 print("Inexpensive")
6 else:
TypeError: '<' not supported between instances of 'str' and 'int'
Let’s re-write the function to return a value instead of priting.
def price_rating(price):
"""Returns whether the price is
considered expensive or inexpensive"""
if price < 100:
return "Inexpensive"
else:
return "Expensive"
price_rating(50)
'Inexpensive'
Here’s another quick example of if statements, and a function you know well.
def abs(x):
"""Returns the absolute value"""
if x < 0:
return -x
else:
return x
abs(-3)
3
Let’s write some checks to test our code more thoroughly.
check(abs(-3) == 3)
check(abs(0) == 0)
check(abs(3) == 3)
Nested if statements#
Instead of just using if
and else
we can used a elif
(contraction for “else if”) to test multiple Boolean conditions in sequence.
def price_rating(price):
"""Returns category for a price."""
if price < 200:
return "Inexpensive"
elif price < 300:
return "Expensive"
elif price < 400:
return "Very Expensive"
else:
return "Outrageous"
price_rating(280)
'Expensive'
check(price_rating(100) == 'Inexpensive')
check(price_rating(250) == 'Expensive')
check(price_rating(500) == 'Outrageous')
We’ll use apply
to add a column to our Monopoly table and help give new players a sense of categorization of inexpensive to expensive properities.
ratings = tiny_monopoly.apply(price_rating, 'Price')
ratings
array(['Expensive', 'Inexpensive', 'Outrageous', 'Inexpensive',
'Expensive', 'Expensive', 'Expensive', 'Expensive', 'Inexpensive',
'Expensive', 'Very Expensive', 'Inexpensive', 'Very Expensive',
'Very Expensive', 'Very Expensive', 'Inexpensive', 'Inexpensive',
'Inexpensive', 'Inexpensive', 'Expensive', 'Inexpensive',
'Inexpensive'], dtype='<U14')
rated_monopoly = tiny_monopoly.with_columns("Cost Rating", ratings)
rated_monopoly
Name | Color | Price | Cost Rating |
---|---|---|---|
Atlantic Avenue | Yellow | 260 | Expensive |
Baltic Avenue | Brown | 60 | Inexpensive |
Boardwalk | Blue | 400 | Outrageous |
Connecticut Avenue | LightBlue | 120 | Inexpensive |
Illinois Avenue | Red | 240 | Expensive |
Indiana Avenue | Red | 220 | Expensive |
Kentucky Avenue | Red | 220 | Expensive |
Marvin Gardens | Yellow | 280 | Expensive |
Mediterranean Avenue | Brown | 60 | Inexpensive |
New York Avenue | Orange | 200 | Expensive |
... (12 rows omitted)
rated_monopoly.group('Cost Rating')
Cost Rating | count |
---|---|
Expensive | 7 |
Inexpensive | 10 |
Outrageous | 1 |
Very Expensive | 4 |
rated_monopoly.where('Cost Rating', are.equal_to('Outrageous'))
Name | Color | Price | Cost Rating |
---|---|---|---|
Boardwalk | Blue | 400 | Outrageous |